Artificial Intelligence (AI) is a branch of computer science that focuses on creating intelligent machines that can perform tasks that typically require human intelligence. These tasks include speech recognition, decision-making, problem-solving, and learning. AI has become increasingly important in today's world due to its potential to revolutionize various industries and improve the quality of life for individuals.
The importance of AI lies in its ability to automate repetitive tasks, analyze large amounts of data quickly and accurately, and make predictions or recommendations based on patterns and trends. This can lead to increased efficiency, productivity, and cost savings in industries such as healthcare, finance, transportation, manufacturing, and customer service. AI also has the potential to solve complex problems that were previously considered unsolvable, such as finding cures for diseases or predicting natural disasters.
Key Takeaways
- AI is a rapidly evolving field that involves teaching machines to perform tasks that typically require human intelligence.
- There are different types of AI, including narrow or weak AI, general or strong AI, and super AI.
- The history of AI dates back to the 1950s and has evolved significantly over the years, with advancements in computing power and data availability.
- AI has numerous benefits and applications, including in healthcare, finance, transportation, and entertainment.
- The components of AI include algorithms, data, and computing power, which work together to enable machines to learn and make decisions.
- Machine learning is a foundational aspect of AI that involves training machines to learn from data and improve their performance over time.
- Deep learning is a subset of machine learning that uses neural networks to enable machines to learn and make decisions based on complex data.
- Natural language processing (NLP) is a branch of AI that focuses on teaching machines to understand and interpret human language.
- Computer vision is another branch of AI that enables machines to interpret and analyze visual data.
- While AI has many benefits, it also poses ethical and societal risks that must be carefully considered and addressed.
Understanding the Different Types of AI
There are three main types of AI: reactive machines, limited memory, and theory of mind. Reactive machines are the most basic form of AI and can only react to specific situations based on pre-programmed rules. They do not have the ability to learn or store past experiences. Limited memory AI systems can learn from past experiences and make decisions based on that knowledge. However, they still lack the ability to understand or predict human behavior. Theory of mind AI is the most advanced form of AI and has the ability to understand human emotions, beliefs, intentions, and desires. This type of AI is still in the early stages of development.
The History of AI and Its Evolution
The concept of AI dates back to ancient times when Greek myths described mechanical beings with human-like intelligence. However, the modern field of AI began in the 1950s with the development of electronic computers. Early pioneers such as Alan Turing and John McCarthy laid the foundation for AI by proposing theories and algorithms for machine intelligence.
In the 1960s and 1970s, AI research experienced a period of rapid growth and optimism. However, this was followed by a period known as the AI winter in the 1980s and 1990s, where funding for AI research dried up due to unrealistic expectations and limited progress. It was not until the late 1990s and early 2000s that AI research began to regain momentum with the development of machine learning algorithms and the availability of large amounts of data.
In recent years, there have been significant advancements in AI, thanks to breakthroughs in deep learning, natural language processing, and computer vision. These advancements have led to the development of AI systems that can outperform humans in tasks such as image recognition, speech recognition, and playing complex games like chess and Go.
The Benefits and Applications of AI
Component | Description | Examples |
---|---|---|
Algorithms | Mathematical formulas and rules that enable machines to learn and make decisions | Decision trees, neural networks, support vector machines |
Data | Information used to train and improve AI models | Images, text, audio, video, sensor data |
Computing Power | The ability of machines to process and analyze large amounts of data quickly | GPUs, TPUs, cloud computing |
AI has a wide range of benefits and applications across various industries. In healthcare, AI can be used to analyze medical images, diagnose diseases, develop personalized treatment plans, and predict patient outcomes. This can lead to faster and more accurate diagnoses, improved patient care, and reduced healthcare costs.
In finance, AI can be used to analyze financial data, detect fraud, make investment decisions, and provide personalized financial advice. This can lead to more efficient financial markets, reduced risk of fraud, and better investment returns for individuals.
In transportation, AI can be used to develop self-driving cars, optimize traffic flow, and improve logistics and supply chain management. This can lead to safer roads, reduced congestion, and more efficient transportation systems.
In manufacturing, AI can be used to automate production processes, optimize inventory management, and improve quality control. This can lead to increased productivity, reduced costs, and improved product quality.
In customer service, AI can be used to develop chatbots that can interact with customers in a natural language and provide personalized recommendations or assistance. This can lead to improved customer satisfaction, reduced wait times, and increased efficiency in customer service operations.
The Components of AI: Algorithms, Data, and Computing Power
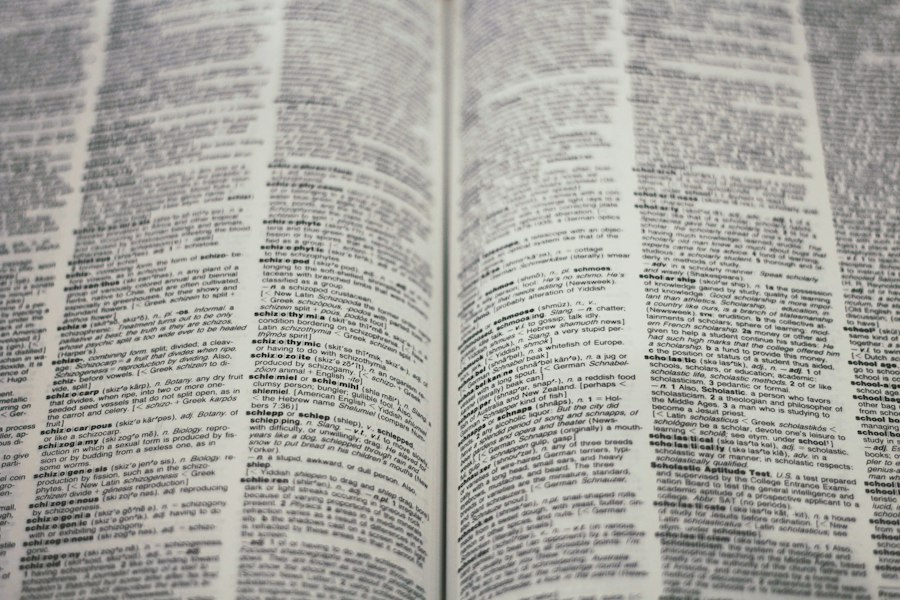
AI consists of three main components: algorithms, data, and computing power. Algorithms are the set of rules or instructions that AI systems use to perform tasks. They determine how the system processes and analyzes data to make decisions or predictions. The choice of algorithms depends on the specific task or problem that the AI system is trying to solve.
Data is the fuel that powers AI systems. AI algorithms require large amounts of data to learn patterns and make accurate predictions or recommendations. The quality and quantity of data are crucial for the success of AI systems. Data can be collected from various sources such as sensors, social media, or user interactions.
Computing power is another important component of A
AI algorithms require significant computational resources to process and analyze large amounts of data quickly and efficiently. Advances in hardware technology, such as the development of graphics processing units (GPUs) and cloud computing, have greatly improved the computing power available for AI applications.
Machine Learning: The Foundation of AI
Machine learning is a subset of AI that focuses on developing algorithms that can learn from data and improve their performance over time without being explicitly programmed. There are three main types of machine learning: supervised learning, unsupervised learning, and reinforcement learning.
Supervised learning involves training a model on labeled data, where the desired output is known. The model learns to make predictions or classifications based on the input features and their corresponding labels. This type of machine learning is commonly used in tasks such as image recognition, speech recognition, and natural language processing.
Unsupervised learning involves training a model on unlabeled data, where the desired output is unknown. The model learns to find patterns or structures in the data without any guidance. This type of machine learning is commonly used in tasks such as clustering, anomaly detection, and dimensionality reduction.
Reinforcement learning involves training a model to make decisions or take actions in an environment to maximize a reward signal. The model learns through trial and error and receives feedback in the form of rewards or penalties. This type of machine learning is commonly used in tasks such as game playing, robotics, and autonomous driving.
Machine learning has a wide range of applications across various industries. In healthcare, machine learning can be used to develop predictive models for disease diagnosis, drug discovery, and personalized medicine. In finance, machine learning can be used to develop trading algorithms, credit scoring models, and fraud detection systems. In transportation, machine learning can be used to develop self-driving cars, traffic prediction models, and route optimization algorithms.
Deep Learning: Advancing the Capabilities of AI
Deep learning is a subset of machine learning that focuses on developing artificial neural networks with multiple layers of interconnected nodes. These networks are inspired by the structure and function of the human brain and are capable of learning complex patterns and representations from data.
Deep learning has revolutionized AI by enabling machines to process and analyze large amounts of unstructured data such as images, videos, and text. This has led to significant advancements in tasks such as image recognition, speech recognition, natural language processing, and autonomous driving.
One of the main advantages of deep learning over traditional machine learning is its ability to automatically learn features or representations from raw data. Traditional machine learning algorithms require manual feature engineering, where domain experts have to manually select and extract relevant features from the data. Deep learning algorithms can automatically learn these features from the data itself, which eliminates the need for manual feature engineering and makes the learning process more efficient.
Natural Language Processing (NLP): Teaching Machines to Understand Human Language
Natural Language Processing (NLP) is a branch of AI that focuses on teaching machines to understand and interpret human language. NLP combines techniques from linguistics, computer science, and machine learning to enable machines to process, analyze, and generate human language.
NLP has a wide range of applications across various industries. In healthcare, NLP can be used to extract information from medical records, analyze patient feedback, and develop conversational agents for patient support. In finance, NLP can be used to analyze news articles, social media posts, and financial reports to make investment decisions or detect market trends. In customer service, NLP can be used to develop chatbots that can understand and respond to customer queries in a natural language.
However, there are several challenges in NLP. One of the main challenges is the ambiguity and complexity of human language. Words and phrases can have multiple meanings depending on the context, which makes it difficult for machines to accurately understand and interpret them. Another challenge is the lack of labeled training data for specific tasks or domains. NLP models require large amounts of labeled data to learn patterns and make accurate predictions or classifications.
Computer Vision: Enabling Machines to See and Interpret Visual Data
Computer vision is a branch of AI that focuses on enabling machines to see and interpret visual data such as images and videos. Computer vision combines techniques from computer science, mathematics, and machine learning to develop algorithms that can analyze and understand visual data.
Computer vision has a wide range of applications across various industries. In healthcare, computer vision can be used to analyze medical images such as X-rays, MRIs, and CT scans to detect diseases or abnormalities. In transportation, computer vision can be used to develop self-driving cars that can recognize traffic signs, pedestrians, and other vehicles. In manufacturing, computer vision can be used to inspect products for defects or quality control.
However, there are several challenges in computer vision. One of the main challenges is the variability and complexity of visual data. Images and videos can have different lighting conditions, viewpoints, scales, and occlusions, which makes it difficult for machines to accurately analyze and interpret them. Another challenge is the lack of annotated training data for specific tasks or domains. Computer vision models require large amounts of annotated data to learn patterns and make accurate predictions or classifications.
Ethics and Risks of AI: Balancing Innovation with Responsibility
While AI has the potential to bring numerous benefits and advancements, it also raises ethical concerns and risks that need to be addressed. One of the main ethical considerations in AI is the potential for bias and discrimination. AI systems are trained on historical data, which may contain biases and prejudices. If these biases are not addressed, AI systems can perpetuate and amplify existing inequalities and discrimination.
Another ethical consideration is the impact of AI on jobs and the economy. AI has the potential to automate many tasks and jobs, which can lead to job displacement and unemployment. It is important to ensure that the benefits of AI are distributed equitably and that workers are provided with opportunities for retraining and upskilling.
There are also risks associated with AI, such as privacy and security concerns. AI systems require large amounts of data to learn and make predictions, which raises concerns about the privacy and security of personal information. It is important to develop robust data protection and cybersecurity measures to mitigate these risks.
In conclusion, AI is a rapidly evolving field that has the potential to revolutionize various industries and improve the quality of life for individuals. Understanding the different types of AI, its history, benefits, components, and applications is crucial for harnessing its full potential. However, it is equally important to address the ethical considerations and risks associated with AI to ensure responsible development and implementation. By balancing innovation with responsibility, we can create a future where AI enhances human capabilities and creates a more sustainable and equitable society.